Why AI Can Push You to Make the Wrong Decision at Work
- Published3 Sep 2024
- Author Simon Spichak
- Source BrainFacts/SfN
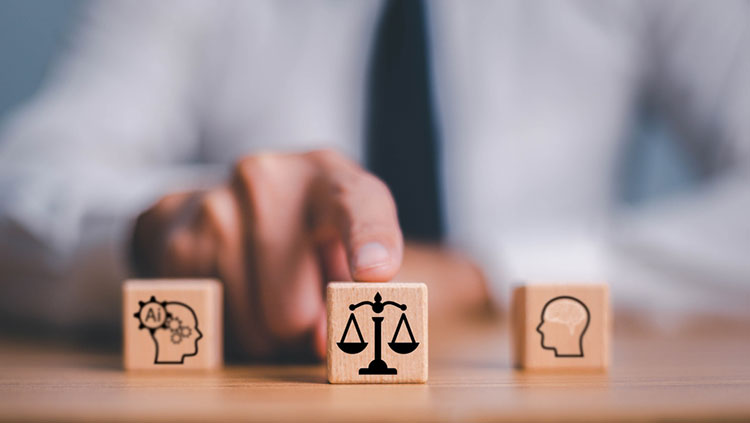
How much do you trust artificial intelligence (AI)?
Chatbots help craft emails and act as customer support. Algorithms help banks decide loan eligibility and assist in reviewing job applications. AI is gaining a foothold in many workplaces, aggregating information and helping people make smarter decisions.
At least that’s how AI is sold. Designed to reduce ambiguity and simplify decisions, AI algorithms can instead lead us to doubt years of expertise and make the wrong decision because of a cognitive shortcut — automation bias. Simply put, it’s our human tendency to reduce our vigilance and oversight when working with machines. This bias can push us to place our faith in the perceived reliability and accuracy of automated systems. And because AI tools are prone to gender and race biases, those can become automated, too.
“People expect the machine [to] actually generate very consistent results,” says Yong Jin Park, a professor at Howard University and faculty associate at Harvard’s Berkman Klein Center for Internet & Society. In reality, Park said AI systems, like ChatGPT, subvert these expectations by providing inconsistent outputs and hallucinations, or incorrect outputs.
Researchers are working to find better ways to design and test AI systems to overcome the dangers of automation bias.
Why Does Automation Bias Occur?
Automation bias is one of many cognitive shortcuts, called heuristics, people use to make faster, more efficient decisions. People might assume automated systems like AI are more accurate, consistent, and reliable than humans and defer to those suggestions.
But AI is far from perfect — racial, gender, and other data biases are built into many algorithms. The result of our reliance on automated systems can be discriminatory, incorrect, and potentially harmful decisions.
“I feel that the [AI] technology has been posited as so advanced, and so capable, that people are afraid to question it,” says Nyalleng Moorosi, senior researcher at the Distributed AI Research Institute. “I think the way they've been marketed is such that people really doubt themselves when faced with a decision: a disagreement between what they think is right, and what the system thinks is right.”
Such automation bias can be deadly. In January 2021, a Sriwijaya Air plane crash killed 62 people. Indonesia’s air transit authority in part blamed the pilots, alongside a faulty autothrottle, for the crash because automation bias prevented them from manually monitoring the airplane.
Automation bias can also impact clinical decisions. Usually, two radiologists are assigned to view a mammogram to assess whether someone has breast cancer. A recent study in Radiology recruited 27 radiologists to test whether an AI assistant could replace the second set of eyes.
“There is a fundamental problem with people who are … going in and designing solutions for areas and applications they do not know enough.”
— Nyalleng Moorosi
The AI assistant conducted a Breast Imaging Reporting and Data System (BI-RADS) assessment on each scan. Researchers knew beforehand which mammograms had cancer but set up the AI to provide an incorrect answer for a subset of the scans. When the AI provided an incorrect result, researchers found inexperienced and moderately experienced radiologists dropped their cancer-detecting accuracy from around 80% to about 22%. Very experienced radiologists’ accuracy dropped from nearly 80% to 45%.
Searching for Solutions to Automation Bias
Despite the integration of AI into our everyday lives, there are relatively few new studies examining ways to reduce the impact of automation bias. Previous tests to mitigate automation bias have delivered limited or mixed success.
One 2023 study in Frontiers in Psychology tested ways to reduce automation bias in hiring tools. 93 study participants were randomly split across various groups. All groups received a computerized dashboard with overview candidate information across three windows: one with a calculated suitability score (either as a percentage or on a five-point scale), another with information about education, abilities, and personality, and a third with a conversation between the applicant and a chatbot. All study participants could access more detailed interfaces on candidate information, which they needed to review to verify the dashboard’s assessment.
Researchers found informing people about error rates and built-in AI biases could mitigate some problems, leading participants to more frequently choose suitable candidates. Providing more data points (i.e. rating candidates based on multiple factors) rather than highly aggregated data (i.e. a score from zero to five) also helped.
However, emphasizing to users that they were responsible for the final hiring decision, even if they’re using an AI tool for assistance, didn't help.
Another 2023 study published in JAMIA tested how to make AI more accurate. Researchers recruited 40 clinicians to look at MRI images of knees with possible anterior cruciate ligament (ACL) tears, and tested their diagnostic accuracy with and without the help of AI.
Although the AI increased accuracy from 87.2% to 96.4%, clinicians still made mistakes. Almost half of those mistakes were attributed to AI automation bias. By understanding how the AI was making errors, researchers adjusted the model, reducing the number of opportunities for AI to bias clinicians.
There’s No Easy Fix for Automation Bias
According to Mar Hicks — professor at the University of Virginia’s School of Data Science — oftentimes, it isn’t worth using AI because of the risks.
Many AI apps are released before they’re tested, safe, and ready. “One surefire way [to prevent automation bias] is to not release what are essentially beta versions of software that isn’t ready for wide release,” says Hicks.
In addition, AI tools should be continually monitored for real world accuracy and utility. “No system should be used in sensitive applications without audit and oversight,” says Moorosi. For example, experts say algorithms, like ones designed to help banks assess loan applicants, should be consistently checked to ensure they aren’t improperly discriminating on factors like race.
“Bias in automated tools can lead to the violation of people’s civil rights,” says Hicks. “At times this may rise to the point of violating the law, for example around issues such as fair treatment in hiring or fair housing. But even when it doesn’t, it can create a variety of other problems that are nonetheless still harmful, and which tend to hurt the groups in society who have the least power.”
Park believes that legal policy must address some of the problems of automation bias. If an employee makes a mistake in a healthcare or business setting that causes harm, is the employee or AI tool to blame? What legal protection or recourse do people have if algorithmic decisions harm them? There aren’t any regulations in the U.S. requiring companies to disclose the use of AI to aid in making certain decisions.
“The problem here is that the law and ethics cannot move quicker than AI.”
— Yong Jin Park
“The problem here is that the law and ethics cannot move quicker than AI,” says Park. He adds that it’s hard to measure the scope of the problems posed by automation bias, and it will be even harder to solve them.
CONTENT PROVIDED BY
BrainFacts/SfN
References
Agarwal, A., Wong-Fannjiang, C., Sussillo, D., Lee, K., & Firat, O. (2018). Hallucinations in Neural Machine Translation. ICLR. https://openreview.net/forum?id=SkxJ-309FQ
Cole, S. (2023). ‘Life or death:’ ai-generated mushroom foraging books are all over amazon. 404 Media. https://www.404media.co/ai-generated-mushroom-foraging-books-amazon/
Dratsch, T., Chen, X., Rezazade Mehrizi, M., Kloeckner, R., Mähringer-Kunz, A., Püsken, M., Baeßler, B., Sauer, S., Maintz, D., & Pinto dos Santos, D. (2023). Automation bias in mammography: The impact of artificial intelligence bi-rads suggestions on reader performance. Radiology, 307(4), e222176. https://doi.org/10.1148/radiol.222176
Hradecky, S. (2021). Crash: Sriwijaya b735 at jakarta on jan 9th 2021, lost height and impacted java sea. Aviation Herald. https://avherald.com/h?article=4e18553c
Kupfer, C., Prassl, R., Fleiß, J., Malin, C., Thalmann, S., & Kubicek, B. (2023). Check the box! How to deal with automation bias in AI-based personnel selection. Frontiers in Psychology, 14, 1118723. https://doi.org/10.3389/fpsyg.2023.1118723
Lyell, D., Magrabi, F., & Coiera, E. (2019). Reduced Verification of Medication Alerts Increases Prescribing Errors. Applied Clinical Informatics, 10(1), 66–76. https://doi.org/10.1055/s-0038-1677009
Mao, F. (2022). Sriwijaya Air crash which killed 62 people blamed on throttle and pilot error. BBC. https://www.bbc.com/news/world-asia-63579988
Notopoulos, K. (2024). Google AI said to put glue in pizza—So I made a pizza with glue and ate it. Business Insider. https://www.businessinsider.com/google-ai-glue-pizza-i-tried-it-2024-5
Park, Y. J. (2023). Let me tell you, ChatGPT-like AI will not change our world. Internet Policy Review. https://policyreview.info/articles/news/let-me-tell-you-chatgpt-ai-will-not-change-our-world/1694
Parasuraman, R., & Manzey, D. H. (2010). Complacency and bias in human use of automation: an attentional integration. Human Factors, 52(3), 381–410. https://doi.org/10.1177/0018720810376055
Sriwijaya B735 at Jakarta on Jan 9th 2021, lost height and impacted Java Sea. (n.d.). Retrieved August 20, 2024, from https://www.aeroinside.com/15231/sriwijaya-b735-at-jakarta-on-jan-9th-2021-lost-height-and-impacted-java-sea
Sundar, S.S., & Kim, J. (2019). Machine Heuristic: When We Trust Computers More than Humans with Our Personal Information. Proceedings of the 2019 CHI Conference on Human Factors in Computing Systems. https://dl.acm.org/doi/abs/10.1145/3290605.3300768
Wang, D.-Y., Ding, J., Sun, A.-L., Liu, S.-G., Jiang, D., Li, N., & Yu, J.-K. (2023). Artificial intelligence suppression as a strategy to mitigate artificial intelligence automation bias. Journal of the American Medical Informatics Association, 30(10), 1684–1692. https://doi.org/10.1093/jamia/ocad118
Also In Tech & the Brain
Trending
Popular articles on BrainFacts.org