Putting Artificial Neural Networks to the Task
- Published31 Jul 2024
- Author Jennifer Michalowski
- Source BrainFacts/SfN
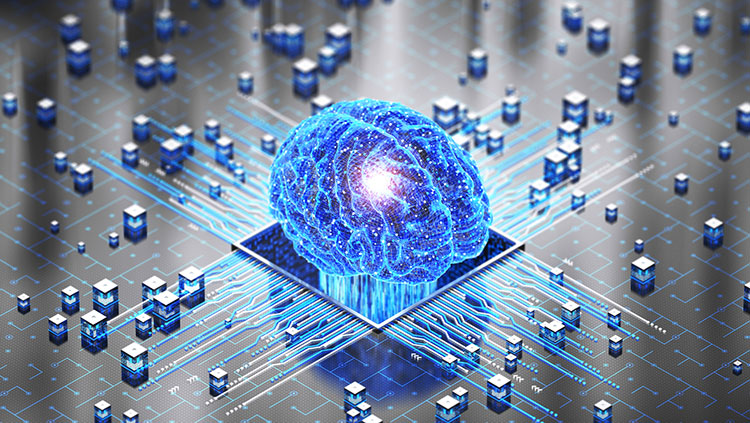
Inspired by the human brain, artificial neural networks are the heart of artificial intelligence. These machine learning algorithms are adept at finding patterns in large volumes of data, allowing them to achieve singularly-focused yet mind-boggling tasks — from managing complex supply chains to diagnosing disease. But unlike the artificial neural networks predicting the words you plan to type or finding the faces in a photograph, brains are not optimized for a single task.
Even though the brains of humans and animals may be more likely to make mistakes than a purpose-built algorithm, they also generate far more complex behaviors. The origins of these complicated and imperfect behaviors are what computational neuroscientist Kanaka Rajan, a faculty member at Harvard’s Kempner Institute, seeks to understand. She is using artificial neural networks to investigate some of the brain’s most sophisticated functions, such as learning, decision-making, and social cognition.
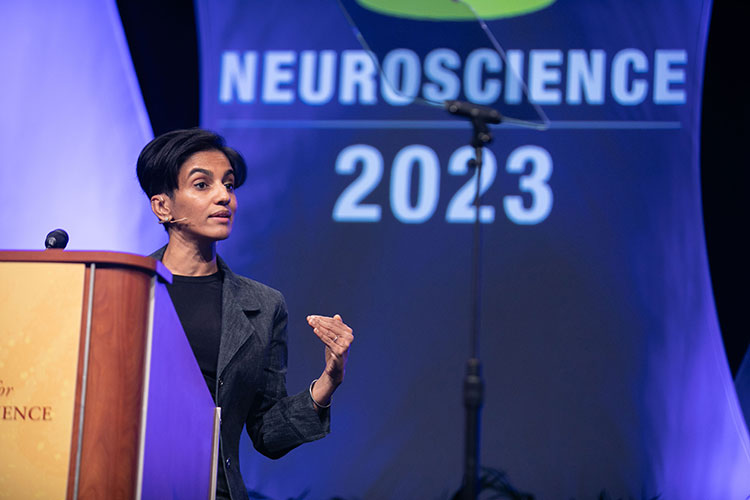
“On the industry side, they have developed AI models by engineering them to do very specific things. For example, a whole bunch of data goes into specialized architectures that can then learn to predict language,” says Rajan, who uses artificial neural networks and other types of models to better understand brains. “But those specialized models can't do a whole lot else.”
The artificial neural networks Rajan builds are computational models developed with data from real animal brains. Like the machine learning algorithms at the heart of most of today’s AI applications, they are made of simple, neuron-like units that organize themselves into information-processing networks when they are trained to do a task. They are different from more traditional models of neural circuits, which deliberately replicate the nervous system’s anatomy or activity.
“Before, if you were building a computational model of the brain, you might take data about what we know about how neurons work and how they're connected and what activity patterns they have, and you would put that together to make this little model of how neurons connect to each other and how they produce their own activity,” says Grace Lindsay, a computational neuroscientist at New York University.
Such models have helped explain many fundamental properties of neural signaling. But unlike neural networks, they don’t transform the input they are given to perform tasks. They can’t produce movement or interpret sensory information, let alone form memories or learn new skills.
Staying On Task
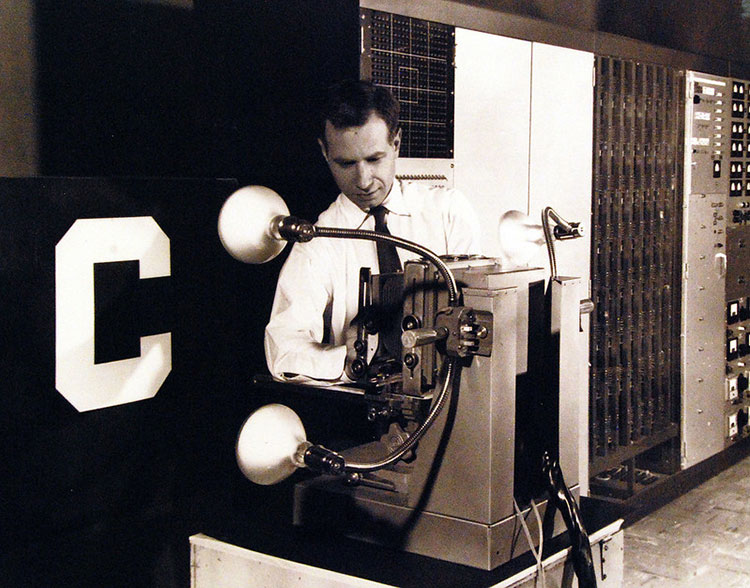
The first trainable artificial neural network was constructed in the 1950s by psychologist Frank Rosenblatt at Cornell University, who built a bulky machine that could learn to discriminate between different visual patterns. Neuroscientists began experimenting with more sophisticated artificial neural networks in the 1980s. But Lindsay says they have emerged as a particularly powerful tool for neuroscience largely within the last decade, fueled by advances in both computing power and AI.
Now that neuroscientists can train artificial neural networks to do things the brain does, researchers can study how those models handle their tasks, in the hopes this will help explain how the brain accomplishes the same thing. Artificial neural networks can learn to recognize visual objects, identify odors, or move muscles. At NYU, Lindsay and her team are using them to study how paying attention helps us learn and perform more successfully.
As new technologies empower neuroscientists to glean more data from their experiments, modelers have been able to incorporate more biological detail into their models and explore increasingly complex brain functions. Less than 20 years ago, neuroscientists who measured neuronal activity were limited to monitoring small clusters of neurons. Today, they can track the activity of thousands of individual cells distributed across an organism’s entire brain, sometimes recording more than a terabyte of data on neural activity in a single session. Experiments may continue for hours or days, with data collected at tens of thousands of time points. At the same time, new technologies like automated video analysis are enabling detailed tracking of animals’ behavior during experiments.
Rajan says models are essential to make sense of this onslaught of data. Fortunately, more data means better models, and the data explosion is enabling Rajan to investigate processes that draw on many parts of the brain. Her artificial neural networks embrace biology’s inherent complexity. Rather than modeling the activity of isolated groups of brain cells, they reflect the impact neurons in one part of the brain can have on the behavior of neurons elsewhere. Just as importantly, these artificial neural networks acknowledge that the brain has not been engineered to excel at one particular task, something many forms of AI do not consider. Rajan wants to understand the ways humans and other animals solve problems, mistakes and all.
Modeling Decision-making
Rajan and her collaborators use data from a variety of organisms, from naked mole rats to humans, to investigate how animals learn, remember, and make decisions. For example, with data Karl Deisseroth’s lab at Stanford University collected from across the brains of zebrafish, Rajan has built artificial neural networks that help explain at the cellular level how an animal’s behavior evolves over time. With Peter Rudebeck at the Icahn School of Medicine at Mount Sinai, Rajan is modeling decision-making circuitry to make predictions about how the brain updates information about potential outcomes.
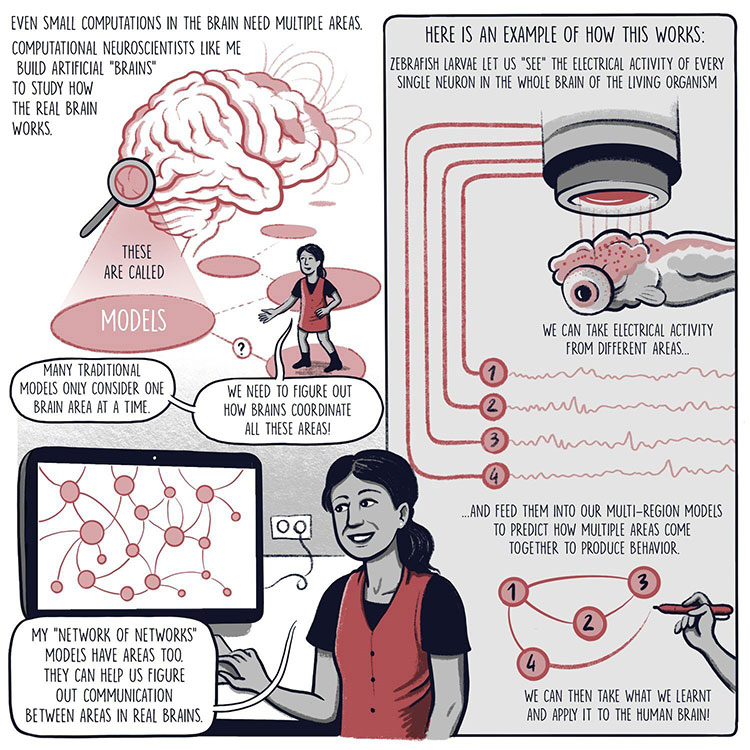
Her models are particularly powerful, Rudebeck says, because they are able to incorporate interactions between many parts of the brain. “Most of the ways that we understand the brain in the moment are just pairwise interactions: area one, area two, and how those talk to each other,” he says. “That’s what makes these models so exciting, because we might get to understand really how our brain works, which is not just two areas talking to each other — it’s five or six or seven [or more].”
Even still, Rajan says, “we may be looking at the wrong scale of things.” Most decisions, she says, are made by small groups of people or animals. So, she and collaborators have begun looking beyond individual brains to study how an animal’s behavior is influenced by the behavior of others.
The plan, she explains, is to develop tiny robots powered by artificial neural networks, trained with data from real animals, then allow small groups of them to communicate and cooperate in lifelike situations, such as foraging for food. The models will inform further experiments with real animals in similarly sized groups, so researchers can learn what drives social dynamics, as well as how interactions change when an individual is affected by conditions such as anxiety or autism. They might even be used to explore social engineering approaches to addressing mental health concerns, Rajan says.
Artificial neural networks won’t explain the brain on their own, Rajan says. A continuous exchange of ideas with experimental biologists is vital. And it won’t take one model, but many. “Biology is not idealized; it’s gnarly,” she says. “I think the way understanding is going to emerge is as a collective. We’re going to need a huge pile of models and theories and discoveries.”
CONTENT PROVIDED BY
BrainFacts/SfN
References
Khaligh-Razavi, S. M., & Kriegeskorte, N. (2014). Deep supervised, but not unsupervised, models may explain IT cortical representation. PLoS computational biology, 10(11), e1003915. https://doi.org/10.1371/journal.pcbi.1003915
Lindsay, G. W., & Miller, K. D. (2018). How biological attention mechanisms improve task performance in a large-scale visual system model. eLife, 7, e38105. https://doi.org/10.7554/eLife.38105
Sussillo, D., Churchland, M. M., Kaufman, M. T., & Shenoy, K. V. (2015). A neural network that finds a naturalistic solution for the production of muscle activity. Nature neuroscience, 18(7), 1025–1033. https://doi.org/10.1038/nn.4042
Wang, P. Y., Sun, Y., Axel, R., Abbott, L. F., & Yang, G. R. (2021). Evolving the olfactory system with machine learning. Neuron, 109(23), 3879–3892.e5. https://doi.org/10.1016/j.neuron.2021.09.010
Yamins, D. L., Hong, H., Cadieu, C. F., Solomon, E. A., Seibert, D., & DiCarlo, J. J. (2014). Performance-optimized hierarchical models predict neural responses in higher visual cortex. Proceedings of the National Academy of Sciences of the United States of America, 111(23), 8619–8624. https://doi.org/10.1073/pnas.1403112111