In the Era of AI, the World’s Best Computers May Be Our Brains
- Published16 Jul 2024
- Author Lauren Drake
- Source BrainFacts/SfN
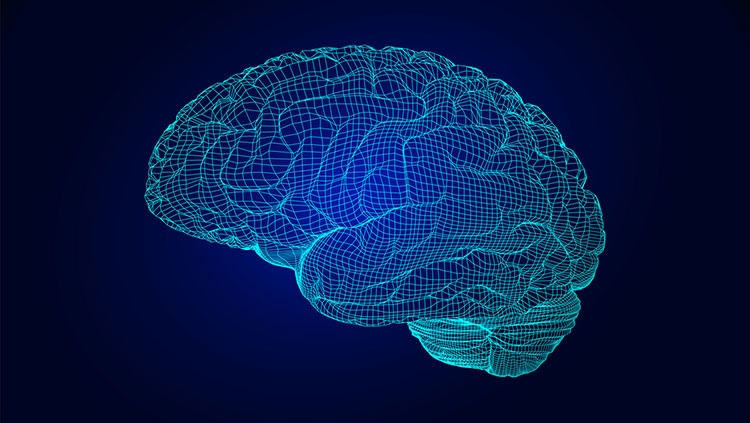
Artificial intelligence is transforming the lives of researchers and patients alike. It helps scientists understand human vision and can translate a person’s thoughts to operate a prosthetic.
The human brain is also a sort of “biological computer,” collecting data about the world through our senses. Modern AI and the human brain both harness the power of processing information. Over the last few decades, researchers have applied the power of computation to understanding how our brains work. The goal: a better understanding of human intelligence and neurological disease. These efforts have forged the rapidly evolving field known as network neuroscience.
“If you don’t know what’s connected to what, how can you ever figure out how the brain is working?” says Olaf Sporns, a computational neuroscientist at Indiana University.
Mapping the Human Brain ‘Connectome’
Network neuroscience is the study of the brain as a system of connections. Researchers use large datasets to study the structure and function of the brain. This research began gaining steam a few decades ago.
But the idea of the brain as a network has been around much longer. Nineteenth-century neuroscientist David Ferrier “almost defines brain networks” in a book he authored, says Cornelis Stam, a neuroscientist at Amsterdam University Medical Center. “He was keenly aware that all these localized functions, they can't exist in isolation, so they have to somehow cooperate.”
A neuroscience textbook may describe the brain as having separate regions controlling specific actions. But this is not the thinking of network neuroscientists. Instead, they study the brain almost like a city transit map. Highly connected intersections in the brain known as “hubs” pass on large amounts of important information.
In a 2005 article, Sporns and colleagues proposed an effort to map these connections in the brain, which he dubbed the human “connectome.” This information would shed light on how the brain creates functional understanding.
“Connectivity has become sort of a way that people interrogate human brains more and more these days,” Sporns says. This has “many applications for understanding brain-behavior relationships, for understanding how the brain functions as a whole in cognition.”
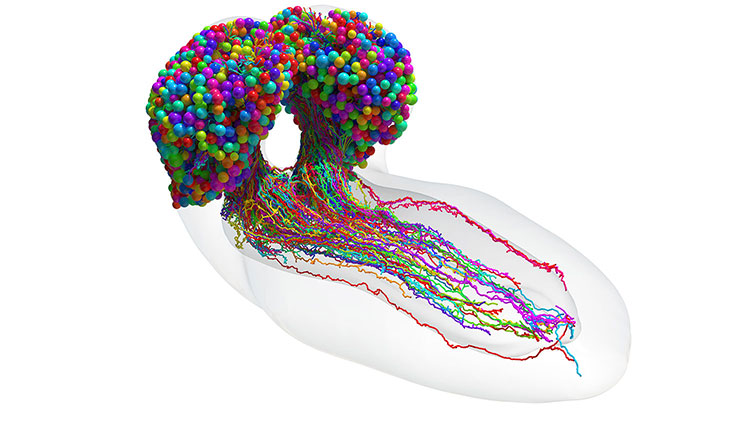
The Power of AI vs. the Economy of the Brain
Understanding these connections and networks can give researchers a better grasp of how the brain is so efficient. For example, a baby may only see a handful of human faces in its first few months of life and can learn to tell them apart. But an AI system needs to be fed thousands of patients’ nocturnal breathing data to predict Parkinson’s disease.
The human brain “is incredibly good at doing some things that computers have a very hard time of doing still,” Sporns says. “And it's very efficient at doing it with very little energy use, with very little training.”
There are several theories for why AI can’t do what the human brain does, says physicist Dani Bassett of the University of Pennsylvania. “One approach is to say that there are different strategies, cognitive strategies that we use, and that those haven't been implemented artificially yet.” Another approach, proposed by Becket Ebitz, a neuroscientist at the University of Montreal, is that there's actually something in the hardware of a human brain that constrains — in a useful way — what computations can and can't be performed, Bassett explains.
“Both of these groups are right, that the distinctions between us and the artificially intelligent systems include cognitive strategies, or software, and physical differences, or hardware,” Bassett says.
AI systems can take a lesson from the human brain’s constraints. It’s small in volume and energy consumption, but still very powerful. And sometimes machine learning algorithms have something to teach us about our brain systems, too.
“Where can we use simple models and have them informed by machine learning?” Bassett asks. “It's actually at that intersection that we'll be able to better understand the unexpected simplicities in complex systems,” Bassett says. “That is, I think, where we can really push forward our understanding and make big, big discoveries.”
Understanding neurological disease
The models of brain networks scientists like Sporns and Bassett develop are not limited to understanding intelligence in the healthy brain. They can provide insights into neurological diseases and disorders we still don’t fully understand.
In conditions like Alzheimer’s disease and epilepsy, damage to brain networks can reroute neurological traffic to other major “hubs,” Stam proposes. Studying these network impairments is a crucial part of understanding and treating these conditions.
“The whole hypothesis is that this overload, or this redirection, actually is the cause of a lot of problems,” Stam says. “If it lasts too long, then you get a cascade. The biggest hubs break down, then the traffic goes to the other hubs, and they also get overloaded.”
“You need problems from neurology, but also hypotheses from neurobiology, and also the mathematical measures and tools, and then it all comes together.”
Stam studies how this overload can be tracked and used as a biomarker, or measurable indication of disease. For example, he and colleagues showed a network model of connectivity in the brain could predict how a patient’s Parkinson’s disease is progressing based on their EEG data.
Human representations of brain networks are inherently imperfect, but they are constantly improving. Bassett is forging new ideas in the study of network control theory. “You ask, what inputs have to be put into the brain in order to push it in a particular direction in some state space?” Bassett says. “How do you move the brain into the state that's healthy versus diseased?” The answers to those questions could have implications for personalized medicine, to determine what kind of stimulation an individual brain might need to return to a healthier state.
And in turn, advances in how we study the brain can feed back into better models of disease. They all contribute to the larger view given by network neuroscience. “You need problems from neurology, but also hypotheses from neurobiology, and also the mathematical measures and tools, and then it all comes together,” Stam says. “I think it's highly interdisciplinary and integrative, but the funny thing is that it is not about one disease. It's not the trick that you apply to one disease, and then it stops. Basically, it's more a way of looking at the brain than a specific solution to a specific problem."
CONTENT PROVIDED BY
BrainFacts/SfN
References
Geraedts, V. J., Boon, L. I., Marinus, J., Gouw, A. A., van Hilten, J. J., Stam, C. J., Tannemaat, M. R., & Contarino, M. F. (2018). Clinical correlates of quantitative EEG in parkinson disease. Neurology, 91(19), 871–883. doi: 10.1212/wnl.0000000000006473
Lynn, C. W., Papadopoulos, L., Kahn, A. E., & Bassett, D. S. (2020). Human information processing in complex networks. Nature Physics, 16(9), 965–973. doi: 10.1038/s41567-020-0924-7
Murphy, K. A., & Bassett, D. S. (2024). Information decomposition in complex systems via machine learning. Proceedings of the National Academy of Sciences, 121(13). doi: 10.1073/pnas.2312988121
Sporns, O., Tononi, G., and Kotter, R. (2005). The Human Connectome: A Structural Description of the
Human Brain. PLoS Comput Biol, 1(4), e42. doi: 10.1371/journal.pcbi.0010042
Winding, M., Pedigo, B. D., Barnes, C. L., Patsolic, H. G., Park, Y., Kazimiers, T., Fushiki, A., Andrade, I. V., Khandelwal, A., Valdes-Aleman, J., Li, F., Randel, N., Barsotti, E., Correia, A., Fetter, R. D., Hartenstein, V., Priebe, C. E., Vogelstein, J. T., Cardona, A., & Zlatic, M. (2023). The connectome of an insect brain. Science (New York, N.Y.), 379(6636), eadd9330. doi: 10.1126/science.add9330
Yang, Y., Yuan, Y., Zhang, G., Wang, H., Chen, Y.-C., Liu, Y., Tarolli, C. G., Crepeau, D., Bukartyk, J., Junna, M. R., Videnovic, A., Ellis, T. D., Lipford, M. C., Dorsey, R., & Katabi, D. (2022). Artificial Intelligence-enabled detection and assessment of parkinson’s disease using nocturnal breathing signals. Nature Medicine, 28(10), 2207–2215. doi: 10.1038/s41591-022-01932-x