To Understand the Brain, You Have to Do the Math
- Published13 Feb 2018
- Reviewed13 Feb 2018
- Author Alexis Wnuk
- Source BrainFacts/SfN
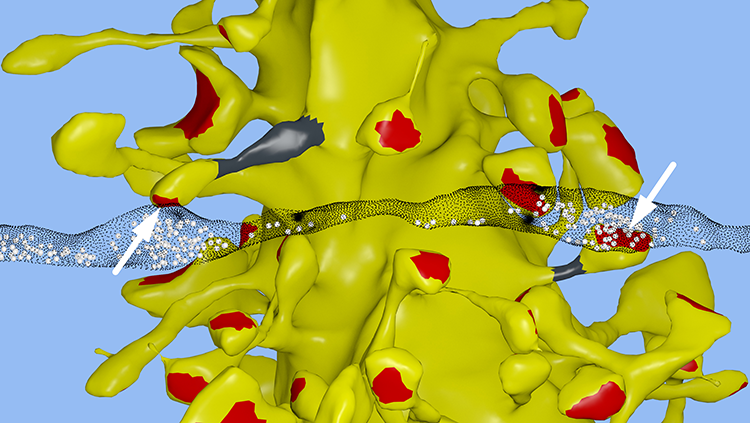
For millennia, humans looked to the night sky and used the stars to guide their way. And, for centuries, astronomers and physicists sought to understand the movements of the universe by gathering data and recording, in great detail, the positions of stars and planets.
Those scientists could predict the movement of these heavenly bodies “even though they didn’t know much about what was driving that motion,” says Alexandre Pouget, who heads the computational cognitive neuroscience laboratory at the University of Geneva. Understanding that, Pouget says, required Isaac Newton and, later, Albert Einstein “to put the universe into equations.
Computational neuroscience is doing the same for the brain — only much, much faster.
Neuroscience is a surprisingly young field. In a matter of decades, the field has grown to include a number of subfields, each of which probes the nervous system from a different angles like genetics, chemistry, molecular biology, anatomy, or behavior.
Most areas of neuroscience are reductionist. They take things apart into smaller pieces and try to explain the mechanisms,” says Terrence Sejnowski, director of the computational neurobiological laboratory and Francis Crick Professor at the Salk Institute and Distinguished Professor at UCSD.
Rather than describing data and observations, computational neuroscience provides a theoretical framework for the study of the brain. “Neuroscience really grew as an experimental field,” says Danielle Bassett, a physicist and neuroscientist at the University of Pennsylvania. “By the time we started thinking about theory in neuroscience we had all of these amazing computational abilities. And so it became called computational neuroscience, when actually I think the goals are theoretical neuroscience.”
Before Einstein, astronomers created elaborate (and accurate) theoretical models of the stars and planets, based on their observations and data. Today, neuroscientists are creating models of how the brain works.
A model makes a prediction about brain activity that can then be tested in an experiment.
For example, you could build a model proposing each area of the brain operates like a pendulum, says Bassett. Areas go from being active to inactive (and back again) in the same way a pendulum swings back and forth. The model makes a prediction about brain activity – areas rhythmically switch on and off — that can then be tested in an experiment. If the prediction doesn’t hold up, the model is amended so it fits the data.
“The hope is that when we have a model that does predict the data well, then the model is a reasonable hypothesis for how the system actually works,” Bassett says.
Bassett leads a collaborative effort at the University of Pennsylvania to build a model of cognitive control — our brain’s ability to override impulses and select behaviors that match our goals. In 2016, her research team received a grant for the project from the National Science Foundation as part of the BRAIN Initiative.
Building and testing models are iterative processes that depend on collaboration between computational and experimental neuroscientists.
Given the increasing complexity we’re facing in neuroscience, it’s becoming clear that we need theoreticians with strong backgrounds in mathematics, physics, and statistics to help us develop more abstract, global models for how the brain works — general models that are not tied to a particular data set or experimental lab,” says Pouget.
“The brain is the most complex computational device we know in the universe,” he says. “And unless we do the math, unless we use mathematical theories, there’s absolutely no way we’re ever going to make sense of it.”
CONTENT PROVIDED BY
BrainFacts/SfN